References
Use of computer vision artificial intelligence technology in dairy cattle
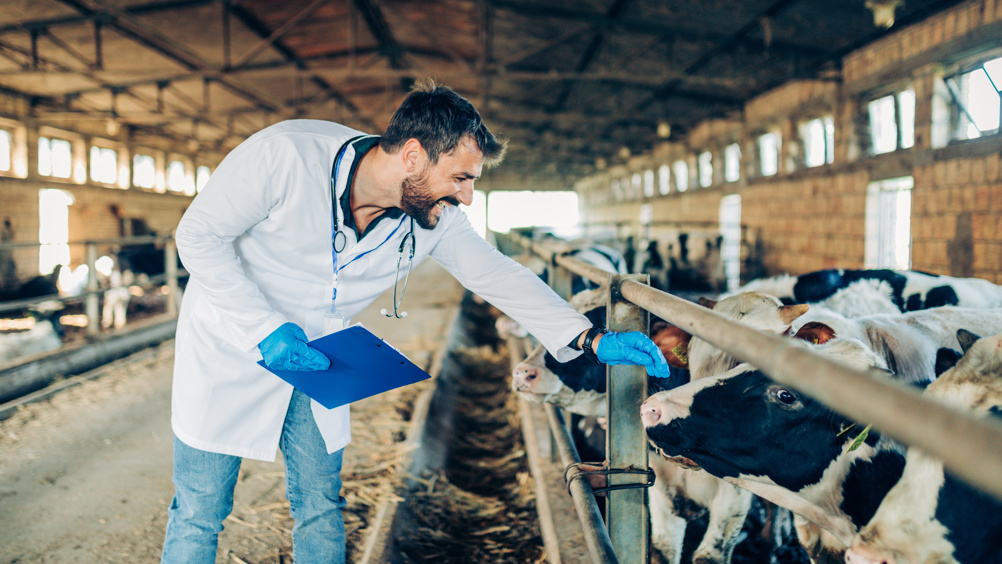
Abstract
Computer vision technology uses artificial intelligence to recognise images and videos of cattle, making predictions on body condition and mobility score, as well as monitoring behaviour on an individual and group level. Precise daily monitoring of body condition in cattle score using computer vision can detect small changes that may not be visible to humans that have been correlated with decreased reproductive performance. However, the systems have typically been found to incorrectly identify animals as in optimal condition where they may be over- or under-conditioned. Mobility scores can be predicted by computer vision and one commercially available system has been validated in field trials that demonstrated an increased sensitivity in identifying animals with painful lesions. Monitoring behaviours such as feeding, standing and time lying down is also a feature of commercially available computer vision systems that can provide long-term objective monitoring, allowing practitioners to identify management areas to improve cow health and production.
Artificial intelligence (AI) can be considered an umbrella term for any system that can analyse vast amounts of data, learn from the information provided and make outcome predictions. AI is not a new concept in dairy herd health monitoring; for example, many sensor technologies use AI to process data on rumination, activity and temperature in real-time and provide health alerts or heat probability (Neethirajan, 2023) to the herd manager on a simple online dashboard. Computer vision is a form of AI that uses algorithms to ‘teach’ a computer model to recognise cattle images and video footage (Tassinari et al, 2021). This can then perform a multitude of tasks normally conducted by humans, such as identifying individual animals from their morphological appearance and recognising key anatomical landmarks that can attribute values for outputs such as body condition and mobility score. As well as identification of individual animals, computer vision models can be trained to recognise different behaviours of cows in a cubicle or free-housed system and generate time budgets for standing, lying down perching and feeding behaviours (Yu et al, 2022), to give producers and their advisors objective long-term data on cow behaviour and identify management factors that may be contributing to poor performance in a herd.
Register now to continue reading
Thank you for visiting UK-VET Companion Animal and reading some of our peer-reviewed content for veterinary professionals. To continue reading this article, please register today.